This thesis proposes and explores the possibilities of a near future wherein a Multi-Modal Large Language Model (or MLLM) known as Publicus is implemented at an urban scale, and trained on data from existing physical sensors, cameras, LiDAR sensors and more within the city, in addition to the standard general knowledge bases used by current Large Language Models. By compositing these pre-existing technologies, in tandem with a network of specialized AI agents, Publicus is able to assist in the creation and upkeep of a digital twin on the scale of an entire city or larger urban condition. It then constantly cross-checks input data from the physical environment with the digital mode to discover any inconsistencies, acting as an immune system to the urban body.
“AI” as we know it today (ChatGPT, MidJourney, Dall-E, Sora) is still in a state of relative infancy, but is rapidly growing. It won’t be long before “AI” disappears from plain sight and popular discourse, and into the underlying structure of our lives. The implications of these technologies upon the world are so vast and interconnected that they can be treated as a hyperobject, and this project aims to isolate and capture moments where this hyperobject intersects or collides at known and recognizable moments within the fabric of urban life, allowing it to be visualized and documented.
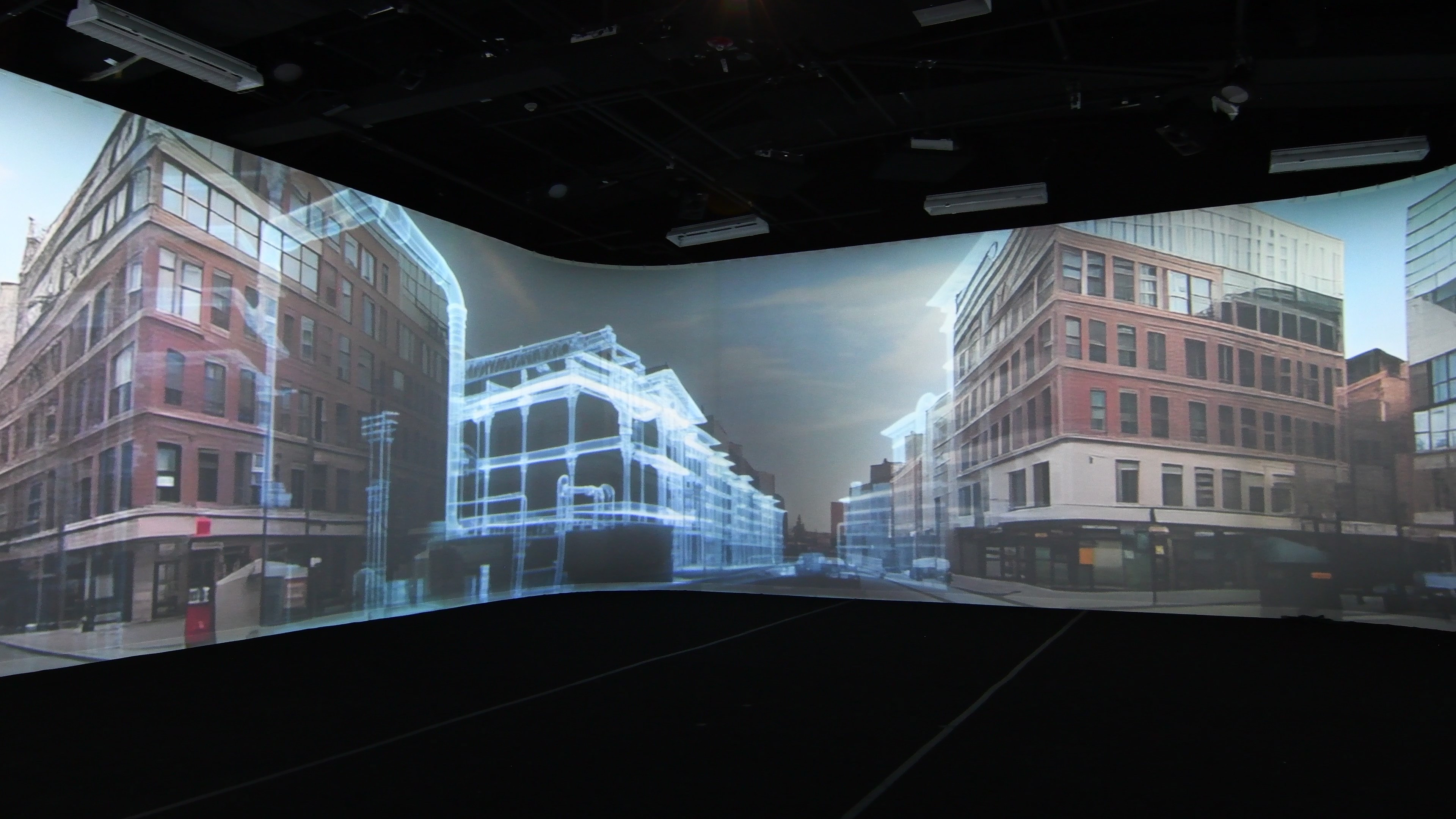
Final thesis being shown in RPI's immersive CRAIVE Lab














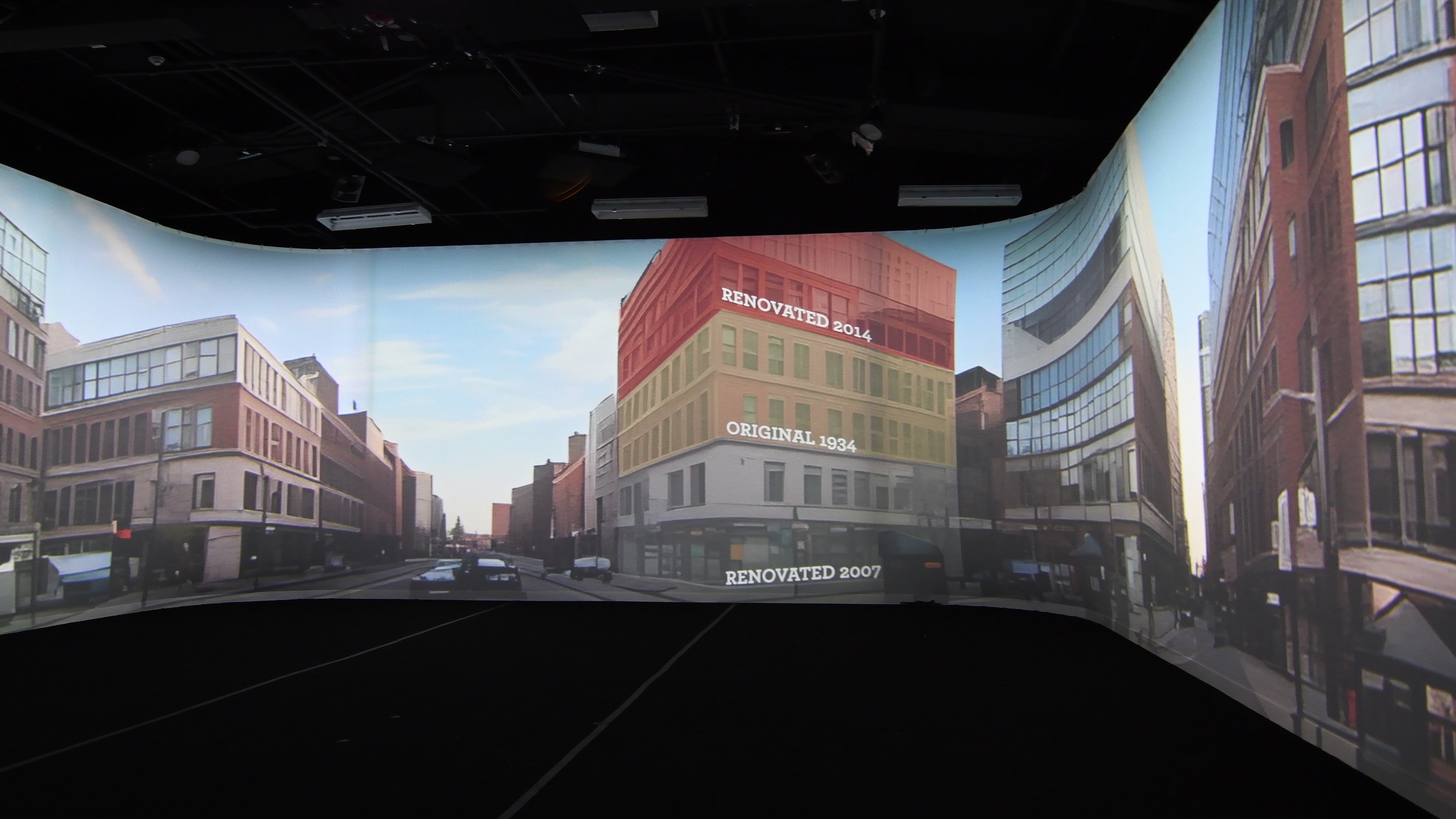